Coronavirus disease 2019 (COVID-19) has spread to nearly every country in the world. While mass vaccination administration has allowed many governments to dismantle restrictions, the constant influx of new variants threatens stability.
Study: Predicting the mutational drivers of future SARS-CoV-2 variants of concern. Image Credit: CROCOTHERY/Shutterstock
Many European countries have introduced new measures to curb transmission and encourage vaccinations, and infections have begun to peak once again. Variants often show worrying features, such as the ability to evade both vaccine-induced and natural immunity, as well as increased transmission.
Most recently, the Omicron variant has ignited fears of overwhelmed hospitals. Most of these variants carry mutations that cause these features. In a study published in Science Translational Medicine, researchers have been trying to create a technique to predict which of these mutations will spread.
The study
The researchers classified any specified fold change in frequency of amino acid mutations across multiple countries as 'spreading' mutations. They tabulated the number of sequences containing the mutation being modeled versus those that did not. A fold change was calculated for each mutation, and mutations with a significant Benjamini-Hochbert adjusted p-value from any country were retained.
The set they then acquired was further filtered, requiring any mutation to have a fold change from a baseline of at least 10.0 in at least one country, a fold change of at least 2.0 across at least three countries, and a minimum global frequency of 0.1% in the later time window.
The sequences used to calculate fold change from baseline and minimum frequency were collected after those used for model training to maintain good practice. This definition was found to capture the expansion of variants of interest (VOIs) and variants of concern (VOCs) globally, as well as several less well-known mutations.
The researchers tested for linkage between all pairs of spreading mutations to ensure that none of the identified mutations were not accumulating independently. They found that fewer than 5% of mutation pairs showed enrichment for co-occurrence at a rate greater than 8-fold.
Following this, the scientists attempted to determine which features of amino acids could be used to predict their spread from baseline. They used angiotensin-converting enzyme 2 (ACE2) binding affinity as the predictor of mutation spread and change in in vitro expression of spike protein mutants.
Binding contributions of known antibody epitopes were also effective at predicting mutation spread, although CD4+ and CD8+ immunogenicity was not helpful. Natural Language Processing (NLP) scores for sequence plausibility were useful. Still, the best feature for predicting spread used Fixed Effects Likelihood (FEL) to test for consistent selection across the branches of a phylogenetic tree.
Epidemiological features were also very useful for predictions, as the variables more directly measure sampled mutation counts. The exponentially weighted mean ranking across multiple epidemiological variables is known as the 'Epi Score.' It is useful for capturing both lineage expansion and recurrent mutation occurring in multiple lineages by convergent evolution.
To ensure this approach would work correctly, the researchers measured the predictive performance of antibody binding scores, revealing the predicted percent contribution of each spike site to antibody affinity. Using this to estimate B cell immunodominance and then taking the maximum of the value across antibodies showed the maximum antibody binding score, significantly increasing this metric's predictiveness. This method was not as effective as examining epidemiological features for predictiveness over the summer of 2021 (when the Delta variant emerged).
Finally, the researchers trained models to predict the spread of mutations using as many previously discussed features as possible, using logistic regression with these features as inputs. They found that the best predictors were epidemiologic features and positive selection features. The full model they developed was comparable to the predictive performance of the Epi Score. This revealed that the top five mutations with the potential to spread were spread across different proteins. In the spike protein, G142 and T951 were most at risk of spreading; in NSP3, it was A1711V; in the nucleocapsid protein, it was Q9L; and in NSP2, it was K81N.
Conclusion
The authors highlight that they have successfully established a working method to predict the spread of mutations and successfully applied it to identify the most likely mutations in future VOCs. They argue that this approach will be incredibly useful in helping to identify the mutations most likely to spread months in advance, allowing research and analysis to begin earlier, potentially resulting in quicker treatments and advice.
- Mayher, et al. 2022. Predicting the mutational drivers of future SARS-CoV-2 variants of concern. Science Translational Medicine. doi: 10.1126/scitranslmed.abk3445 https://www.science.org/doi/10.1126/scitranslmed.abk3445
Posted in: Medical Science News | Medical Research News | Disease/Infection News
Tags: ACE2, Amino Acid, Angiotensin, Angiotensin-Converting Enzyme 2, Antibodies, Antibody, B Cell, binding affinity, CD4, Cell, Coronavirus, Coronavirus Disease COVID-19, covid-19, Enzyme, Evolution, Frequency, immunity, in vitro, Language, Medicine, Mutation, Omicron, Protein, Research, SARS, SARS-CoV-2, Spike Protein, Vaccine
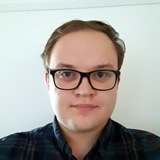
Written by
Sam Hancock
Sam completed his MSci in Genetics at the University of Nottingham in 2019, fuelled initially by an interest in genetic ageing. As part of his degree, he also investigated the role of rnh genes in originless replication in archaea.
Source: Read Full Article